Using AI in the Development of New Ophthalmic Treatment
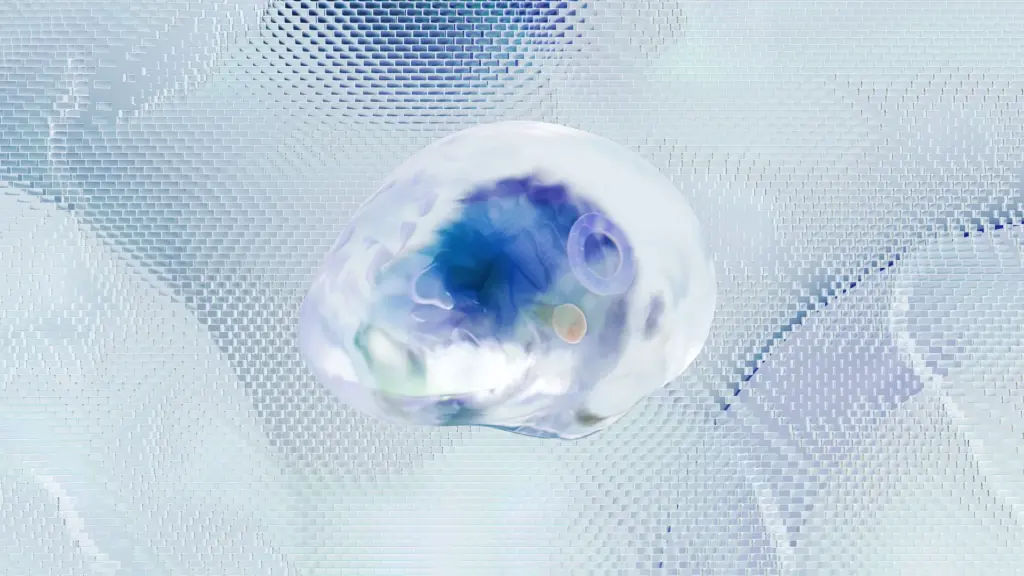
The following is a transcript of a Q&A interview on the use of AI in ophthalmology clinical trials.
What are the specific opportunities and benefits of artificial intelligence (AI) in clinical trials?
In recent years, the big shift that we’re seeing in clinical trials is that the typical way to think about clinical trials was all around FDA regulations. So FDA approval going for FDA-approved endpoints. That certainly is important for a number of reasons. But in addition to that, we’re looking at endpoints that are going to affect real-world outcomes for patients, for payer access, and for physician and patient uptake, right? What we’ve seen, what we’ve had some experience with, or a number of projects where you get an FDA approval, but the market access might not be great because of the design of the clinical trials. And so that’s one of the key characteristics that we’re now thinking about in clinical trials as we develop new therapeutics.
What is the vision of Lexitas Pharma Services and the company’s main functions?
So the vision for Lexitas is to be a clinical development partner for ophthalmic companies. We typically partner with any size of company that’s developing in ophthalmology, whether it’s a tiny biotech with one or two people all the way up to large pharmaceutical companies. And what we do is we offer really specific solutions for how to get their medication from preclinical into an approved status. So that’s what we’ve specialize in. We do a lot of regulatory work, we do a lot of medical strategy work, we do a lot of clinical operations, biostatistics, things like that. So that’s really the core of what Lexitas does.
What is the role of AI in the pharmaceutical or medical device development process to help create new medicines?
On the actual drug discovery side, I know that there are many people who are using artificial intelligence to help target specific receptors, specific mechanisms within the cellular biology. But at Lexitas, we are further downstream than that when we’re running clinical trials. And so the way that artificial intelligence affects us is really around several different points. The first point is around the development of inclusion-exclusion criteria, right? So one of the things that we always are trying to do, one of the key principles of trial design, is getting the right medicines to the right patient at the right time. So we want to capture the patient at the right moment in their pathology, where we expect the medicine to have the maximum benefit. And we want to capture patients who actually have the right cellular biology to respond to the mechanism of action. And there’s sometimes there’s a discord between the phenotype. What the physician can actually see on evaluation and what’s actually happening.
A good example of that is diabetic eye disease. Diabetics have very complicated pathobiology, and in the eye, some of it’s VEGF-mediated, or vascular endothelial growth factor-mediated, where you get leakage of the blood vessels, growth of new blood vessels. And another aspect of it is inflammatory, where you get breakdown of the blood-retinal barrier. You get a lot of sequelae from chronic inflammation.
And one of the things that we’ve used artificial intelligence for, we currently are developing is to look at patients’ characteristics at baseline. Whether it’s imaging characteristics or patient history, and see if the computer can predict the patients that are going to respond more to anti-inflammatories or respond more to anti-VEGF-mediated pathways. And that, the way you develop that, the way we’re developing that is we’re taking a real-world data set of patients who are treated with either VEGF or steroid, and we’re looking at that response. And so we’re feeding the baseline information into the computer, and then we’re also feeding in whether or not that patient responded. And it’s a very binary thing, right? We literally are just telling the computer, the patient responded or the patient didn’t respond, and we’re asking the computer if they can develop an algorithm to predict the patients who respond and don’t respond based off all of the initial criteria. And so that’s the first way that we’re using artificial intelligence is to try our best to pick out the right patients to include in trials.
The next way we’re using artificial intelligence is on the other end of the trial, where we’re evaluating the effect of the drug, right? So obviously, the two things that we care about are, is the drug safe, and is the drug efficacious? And so we use artificial intelligence to help us really with both of those. So we have the computer look at all of the patients who were treated and try to identify commonalities between the treated patients,
I’ll give you a specific example. So we use something called OCT, optical coherence tomography. It’s a way to get high-resolution cross-sectional scans through the retina at a resolution of about eight, an axial resolution of about eight microns or so. And it gives you a number about 512 slices through the retina. And we’re using artificial intelligence right now to interpolate those results to the remainder of the retina to give us a volume of fluid in diabetics. And so you have swelling in diabetics. Typically, the old way we would do it is we would just measure the thickness of the retina in any one slice, right? We call that central retinal thickness. What we’re doing now is we’re actually getting a volumetric measurement in microliters of what the volume of fluid is, which is a fantastic advance.
The other advances that are using artificial intelligence are really around adaptive optics. So these are the ability to see individual photoreceptors and evaluate those. And so the deep learning algorithms are a critical piece to this. And there are a number of companies, Retina AI, Voxeleron, there’s a number of computer science companies that are honing in on this and have been great partners for us. Ophthalytics is another one that are doing great work in the field. So those are the two ways that we typically use artificial intelligence is to select the right patients for the right trials, and then also to gain further insights into the safety and efficacy of the medicines we’re testing.
I’m just wondering, when you’re using AI perhaps specifically around patient selection, are there any particular strategies you think might be applicable in some cases, better than others? Are there different ways in which this AI can be leveraged in that respect?
Yeah, absolutely. The different buckets that we put in, number one is an imaging bucket, right? So the images, ophthalmology is a very image-intensive field. Whether it’s photographs of the eye or OCTs that I mentioned, or fluorescein angiograms, there’s a lot of different ways to image the eye. And those images typically rely on pattern recognition from human, right? So that’s the traditional way that we do it.
And we are augmenting that pattern recognition by humans with pattern recognition by the computer. And so that’s one bucket is with image recognition. And that’s really pretty simple. I mean, it literally is feeding jpeg images that are into a computer with certain classifiers. And really, you know, there are some big data sets. We’ve trained models on as few as 70 patients, so we don’t have to have a massive data set to get a good model. Now, I will tell you that all of the computer scientists we work with will tell you that obviously, the more patients you have, the better the model will be. But, you know, we certainly have done it with smaller data sets as well.
The second bucket is around genetics. And, you know, genetics are an interesting field, right? Because there are certain genetic mutations that have been in class, right? So you actually get the report back from the geneticist that says, “Hey, here’s the codon, here’s the codon alteration, and this is known to be pathogenic.”
But you have a whole subset of genetic anomalies that we aren’t sure if they’re pathogenic or not. And they can happen in a lot of different combination. And you can get a lot of data where, you know, essentially the geneticist will tell you, “Hey, this is possibly pathogenic, but we don’t know.”
And so using the computer to look through all of that data and try to pick out patterns amongst patients is really going to be on the forefront of what we’re doing from a genetic standpoint, particularly in eye disease. Because the world of inherited retinal degeneration is a very ripe field for this kind of work. You know, the first gene therapy was developed by Spark Therapeutics for RPE65, which is an eye condition. And so gene therapy in the eye is, it is quite a big field.
And then the third bucket, I would say, is looking at the overall patient picture, right? There are partners of ours out there that are working with mining the EMR, right? So the algorithm can actually go into the electronic medical record and look at characteristics of patients and use that to, use that to try to pick out patterns about how the patients are responding, pick out patients that meet inclusion-exclusion criteria, and produce essentially a list of patients for the clinical trial, potential patients. And so using the algorithms to look into the EMRs is incredibly powerful, particularly if you can get an EMR that’s cloud-based and deployed across the country or across the globe. It allows you to really select out where you’re going to open clinical trial sites.
We’ve used technology like this to say, “Okay, we need a site, and, you know, we need to use London, New York, and Miami, you know, for our sites because that’s where the patients are, things like that. So that’s a really powerful way to identify patients, is looking at the EMR at the entire patient picture to find patients, and really to look for patterns in the EMR for patients who respond to different treatments and things like that based on their criteria that have been entered.
I’m just thinking, we’ve already started looking, I suppose, more deeply in its implication, the implication of AI use within ophthalmology clinical trials there, and some solutions to the existing obstacles, I suppose. You’ve outlined imaging, genetics, patient selection. Presumably it’s not just an improved accuracy and efficiency of the process, but inherent to that efficiency is a time saving. What are the general time implications or time-saving implications of using AI in this way?
Oh, absolutely. So two aspects to it, right? Number one is the more you know about your drug, the more you know about how your drug affects people, right, the easier it is to power your study. So in an ideal scenario, if you’re able to use that AI to either, to either pick out patients that are more likely to respond or to have more refined endpoints, then you can decrease the number of patients you need to enroll, which is a fantastic thing, right? Because you don’t, you want to keep your trials to the minimum size necessary to power the trial to show safety and efficacy. And that’s just the core principle of clinical work.
The second way that it’s affecting what we do at Lexitas, which is enrollment in clinical trials, which is also directly related to the timelines, right? So slow enrollment is the number one reason why clinical trials run long. And in the industry average is that 80% of clinical trials across the globe and across therapeutic area do not enroll as expected. They enroll slower than expected. At Lexitas, we actually are the exact opposite. So we’re enrolling ahead of target or at target on >80% of our trials right now. And we do that based on a lot of aspects outside of artificial intelligence. We do a lot on relationships. We do it a lot on the details of protocols, making sure that they’re achievable by the sites and the patients.
But we also do use a lot of data to make sure that when we go to a place that we’re finding the right patients, right? A good example of that is a pediatric rare disease, inherited retinal degeneration study that we’re doing where these patients are, you know, a handful across the globe and being able to identify these patients relies deeply on computer algorithms finding where these genetic mutations exist, tracking them back to where the patients are, and then offering these children and their families treatment if they wish to participate.
What are the risks or potential challenges that need to be recognized and mitigated for, or any kind of potential limitations that need to be overcome and therefore smoothed out as you’re applying this? Presumably, we’re still to the relatively early stage of using this technology?
Absolutely. I mean, just like any technology in the early stage, we’re extremely careful with how we deploy it, right? Particularly with stakes as high as they are in clinical development with patients getting new medicines, it’s critically important that we go deliberately, we go knowingly, and we, we are very intentional about how we deploy it. And so, it’s something we take very seriously.
What we don’t want to have happen is we don’t want to have any sort of, you know, unknown consequence of the AI, right? We don’t want to end up excluding different people populations from trials unnecessarily, right? People that may have benefited from the drug. That’s the other side of trying to pick out the right patient is if we get too narrow, then you’ve got a drug that truly hasn’t been studied like it should. And so that’s something that we take very seriously. The whole diversity and inclusion aspect of clinical trials is a big deal, and it’s something we’re very intentional about. And so that’s the aspect that I’m hitting on there.
The other part of it is sometimes we don’t fully know the characteristics of the algorithm, particularly if it’s looking at imaging data, right? So it picks out patterns, but the computer doesn’t always tell us exactly what it’s picking out. And so there’s always a chance that it’s looking at essentially a false positive, right? The computer is looking at something to pick out a pattern that’s not a true biologic, you know, pathobiologic correlate with the disease. And so we have to be very careful with that as well.
There are also unknowns based on, on the FDA regulatory pathway. I know that Scott Gottlieb, the former FDA commissioner, has published recently a good amount on the AI frameworks at the US FDA, and I know that the FDA itself has been looking hard at this and has been publishing some draft guidance around this. But it’s an evolving regulatory world as far as, as far as use of AI in clinical trials go.
And also the real-world implications, right? So in a clinical trial, we can closely control trials with our algorithms, but once the drug is approved and it’s being used by physicians and patients around the world, you know, they may or may not have access to that algorithm as particularly in less developed countries. It’s going to be a paradigm shift to utilise this in real-world clinical trial, real-world medicine.
And so those are all considerations that we have to take. And balance, right? It is a tight balance because those are some pretty significant challenges to overcome, but the advantages of being able to get the right medicine to the right patient at the right time, I think, outweigh that. And we just have to work through the challenges in a really deliberate and intentional way as we all navigate this new field, these new possibilities.
When thinking about AI even generally, so often the focus is on how do we essentially smooth out and limit the biases that might be inherent, even just implicitly or even accidentally?
It is a very relevant consideration. And I can tell you, I can tell you how we approach it, and I think there are numerous ways to approach it, but the number one way we approach it is, is that all of our clinical trials are still being run with at least some studies in the clinical development pathway that do not utilize artificial intelligence or deep learning algorithms. So we still do have. You know, every clinical development program, right, is numerous trials. Most of the time, there’s anywhere from, you know, five to 10 trials before a drug gets approved. And at least some of those trials are either safety trials or early-stage trials or late-stage trials that are enrolling very diverse populations, right?
And so the data exists, and so what you can do on the back end is you can compare, right? So you can look at the data from your traditional trials, you can look at the data from the enhanced trials with different algorithms, and you can evaluate, right, what’s happening. And so we haven’t gotten to the point yet, and I still think it’ll be a while before we get to this point where we are developing, doing an entire development program based on some aspect of a computer algorithm. We still are, I mean, the way it’s working now is the early-stage trials are being done, essentially, in broad populations without computer intelligence. And then the later-stage trials are being honed based on models created through that process, so you’re gathering the input data for the model from the earlier trials. And so that does help protect you against bias, and that’s how we’re doing it at Lexitas. And I’m sure that there’s a lot of different ways of detecting bias from a computer science aspect of it that are outside of, outside of my scope.
Let’s focus on perhaps on regulating the use of AI-informed efficacy in effectiveness estimates. What do you think some of the key considerations and hot topics around the use of estimates of efficacy being informed by artificial intelligence?
Currently, artificial intelligence is being used, so when you’re evaluating efficacy, when you’re looking at endpoint evaluation, the number one thing we care about is improvement in patient quality of life, right? Functional life. And most of the artificial intelligence endpoints are around structural changes. So what, you know, changes in the actual anatomy. And so I think one of the good checks and balances here, and one of the good things that we need to all keep in mind is that at the end of the day, the thing that matters is not what the computer algorithm pops out, it’s how the patients respond to the medicine and the day-to-day difference in their lives that it makes.
And that’s the core, that’s the core of clinical development. And I think that those are going to continue to be primary endpoints, right? With the artificial intelligence-driven algorithms being supplemental endpoints help us understand why, you know, the science behind it, why is this, what exactly is this drug doing that’s leading to a positive impact in this patient’s life? So that’s how I see it, and that’s honestly how I look at my trials is, yeah, it’s wonderful, and it’s so important to understand the structural anatomical changes to the pathobiology, but at the end of the day, it’s kind of, I mean, it’s as simple as the so what matter, right? So the question is, you know, you’ve modified this disease, so what does that mean for the patient?
How do you feel artificial intelligence presence might alter approaches to evidence synthesis from trial data? Are there any examples you can perhaps share in that respect?
Yeah, well, I can tell you there’s some ongoing, there’s some ongoing efforts within ophthalmology particularly that get at this, right? So in ophthalmology, we’re privileged and blessed to have a massive registry of clinical data that’s kept by the American Academy of Ophthalmology. It’s called the IRIS Registry. And it’s a database of, at this point, likely hundreds of thousands of patients that can be mined, right? And I think that that volume of big data sets in the real-world are just a gold mine for computer algorithms to go through. And the key advance to that registry that quite hasn’t quite happened yet but is on the horizon is going to be the addition of clinical images, right? So OCT images from the photography into a database like that. Retina Consultants of America is a great example. They are a group of 200 retina specialists in the United States that just put all their images into the cloud. And Retina AI is a company that specializes in artificial intelligence for the retina, has just announced a partnership with them to evaluate their images. And so that’s a real example in the recent, in the recent news of real-world evaluation of images using computer algorithms.
I think it’s going to be more and more. I think, you know, we are just implementing machine learning, deep learning into clinical trials. I think that the next logical step is going to be implementing it into everyday practice. And it’s going to be an amazing tool for physicians to have. You know, I’m envisioning a day, like, it would be fantastic to envision a day where you can truly, you can truly predict a patient’s course of disease and whether or not a drug can actually modify it. So rather than just saying, “Ms. Jones, you have macular degeneration, you may or may not lose vision in the future,” I would love to be able to say with a lot of certainty exactly what’s going to happen, and if we treat you, we would expect you to improve, or it’s not as likely to improve. And so those are some of the real-world things that I see when I see my patients that I wish I could answer with more certainty.
AI could be used increasingly to answer well-specified questions, which may otherwise take more resource and other considerations to undertake. It may speed up and perhaps make that a more accurate endeavor. Could AI be used not just to answer the questions that exist but actually to devise the right set of next questions?
That’s an interesting question, and I think the answer is yes, and I think that the extrapolation on that is that artificial intelligence is going to give us new information about medicines, right? And how they impact people. And that new information will create a whole new set of questions. So as we continue to learn more about disease pathology, responses to medicines on imaging, on genetics for gene therapies, things like that, I think there’s going to be all kinds of questions that arise from that.
How do you feel that the relationship between AI and health economics might influence strategies for both pricing and reimbursement?
So if you can increase the efficacy of a drug, right? So whether it’s by better design of the drug in the drug discovery process using AI, whether it’s refinement of the patient population on who gets the drug or when they get the drug in their diseased pathology, that is going to be a big thing. So who or when they receive the drug, and from a health economic standpoint, if you can reduce the unnecessary exposure, that is going to make a huge impact on pricing, on expenditure, and on unnecessary treatments. So I think that there’s a direct correlation right there.
Could you outline your thoughts on the future prospects of AI in pharmaceutical development? For example, what sort of transformation might we expect in years to come?
Oh, we can expect it to touch every aspect. So we talked about drug discovery, it’s going to allow us to have more targeted medicines. It’s going to allow us to be more efficient with what we, with what we do in clinical operations and clinical development. And it’s going to help us, it’s going to help us in the real-world get the right medicines to the right patients. So it’s really going to be transformational.
Our In Focus newsletter is published once a month and has thousands of subscribers. To help you keep in touch, you can subscribe here.