AI Helps Identify Patients Likely to Respond to Treatment During Ophthalmology Clinical Trials
Transcript
Hello, my name is George Magrath and I’m the CEO at Lexitas Pharma Services. I’m also an ophthalmologist.
And I’d like to talk to you today about the way we’re using machine learning and artificial intelligence in clinical trial work that we do in particular dry AMD but also in other indications as well.
So what we really care about is really taking all of the advances that have happened in the world of machine learning and AI and really applying it to clinical trial work in ophthalmology.
And there’s three different things that we’re thinking about doing that we think will really bring this into the into our world and really improve our ability to get great medicines to patients.
The first is that we want to create an automated algorithm to detect a degree of disease so what we would do is we would light the computer to be able to look at some of the images and tell us whether a patient has early, intermediate or advanced dry AMD or any other conditions.
You know, if they have diabetic macular edema or if they have a renal vein occlusion or whatever the pathology may be.
So the first specific aim that we’ve had that I’ll show data on is that is really taking the OCT scan looking to see if the computer can predict what disease the patient has.
The second thing we’re going to do is we’re going to look at whether or not we can predict the patient’s response to treatment based on an OCT scan.
And the way we do this is really to create a data set of patients who did respond and patients who didn’t respond and then we asked the machine if it can create an algorithm to pick out characteristics at baseline that may predict whether or not a patient will respond or not respond.
And so that goes into the third one which is really to use that algorithm to select patients for clinical trial use based on the mechanism of action that they’re most likely to respond to.
We feel like every patient is a unique situation, every patient has a personalized approach and we really want to bring that in.
Disease pathophysiology is so complex. And we know this from how we treat patients now that there are multiple treatments for most diseases where if one mechanism isn’t totally effective we switch to a different and try that.
The example would be in diabetic macular edema where we sometimes take patients and start them down the anti-VEGF route and then switch over more to an anti-inflammatory route if need be.
So we’re trying to predict that up front so you will know that over the past decade or so really, there’s been a revolution in in deep learning, machine learning and artificial intelligence.
And really over the past few years there’s been a lot of work done in dry AMD and also in other indications really to grade disease, to identify disease and be able to take that into the clinical practice to see if the computer can pick out disease a little better than we can with our naked eye.
So this is important in clinical trial work. So one of the problems particular to dry AMD is worried about the progression. So some patients with dry AMD will progress and these are the patients that you want in the clinical trials.
These are the patients that are at risk for losing vision these are the patients that need to be treated.
There are a lot of patients though that will not progress and those patients will be just fine. You know, they’ll live with a mild amount of macular degeneration that’s not clinically significant for their life and they don’t need to be enrolled and put at risk in clinical trials or with other treatments.
So if we are able to pick out patients that are at high risk for progression it does two things: Number one, it doesn’t expose patients who don’t need the medicine to any risk of the trial.
Number two is that it allows the trial to be able to have a better [inaudible]. So it’ll be in the trial, you’ll need less patience to show a treatment effect because you’ll be looking at patients that otherwise would have progressed.
So it’s a win-win all the way around if you can pick out the right patients for the right trial at the right time, and that’s exactly what we’re trying to do.
So a case example for what we’ve already done in DME so this is in diabetic macular edema around whether or not the computer can detect a disease from a scan.
So this is step one. We took 6725 OCTs and we manually annotated them, so the manual annotation was either that DME was present or DME was absent. It was as simple as that just one binary measurement point.
Then we split the data. 80 of the scans were used to train and validate the computer algorithm, 20 percent were held as a test set. So with the eighty percent training set, we then had the computer work its magic.
And we did a resnet 50 encoder using a supervised contrasted learning algorithm to identify whether or not the patients were DME or not DME.
And then after that, the computer had created its algorithm, we used the twenty percent of images remaining to test that algorithm. We don’t know what the algorithm was looking at in the images.
All we’d asked the computer to do was to train itself on whether or not the patient had DME or not and we didn’t tell it where to look or anything like that. We literally gave it the jpeg image from the OCT and this is what we found.
So the train accuracy was 99.6%. The test accuracy was 99.4%. And of that test set of 1345 OCTs, you see that there was a false positive rate of six patients and a false negative rate of two patients.
Which is incredibly accurate for a model. So this was really encouraging to us that this machine could really pick out patients who have diabetic macular edema versus patients who don’t.
This same model, same thought process concern is being used for dry AMD and that’s currently what we’re undertaking.
And it can be used to cross different indications. This is really a platform. So the next step is really to see if it’s possible to predict disease.
And what I really want to do is take this same algorithm, instead of labeling the images “no DME” or “DME”, what you do is you take a set of images and you say these two images represent response, these two images represent no response, and you run it through the same testing sequence that we talked about earlier.
And the idea is to see whether or not you can really correlate the baseline OCT scan with whether or not that treatment will cause an effect.
Now, once that’s built it can be refined by trying to correlate it with visual acuity data and other functional endpoints.
So you can put as much data into the baseline as you want where you can add in genetics, you can add in whether or not they have complement age defect or whatever the defect is of interest you.
You can also add in on size of geographic atrophy, all these other kind of things that you’d want to look at so you can really create an algorithm that’s not just the image but the entire inclusion criteria to see who’s going to be a responder and who’s not.
And a portion of this has been done by this group that’s referenced here and they did really remarkable work on this where they predicted visual function from automatically quantified OCT biomarkers.
And this is a quite a remarkable paper where they were able to show that that certain characteristics on the OCT are more related with vision loss than others.
And so it really was great work that just came out so there are a number of groups that are working on similar projects like this. But I think that this is the future of how we develop medicines because it truly allows you to find patients that are likely benefit likely to benefit from a certain treatment.
So these algorithms, as I mentioned at the beginning, the Holy Grail, is to be able to really provide personalized medicine by selecting out patients who really are the ones who are likely to respond to the treatment and likely need the treatment.
So you’re really excluding patients who may not respond to the treatment. So patients who we don’t think would get better with medicine, or the patients who we don’t think are going to progress and aren’t going to really have that much of a problem where they need to be exposed to the risk of a clinical trial.
Amazing information that physicians can use when making uh decisions both in the clinical trial setting and in the real world setting.
And with that, I’d like to conclude this presentation. We certainly are excited to talk to you. If you have any questions about this please contact us.
This is an area of passion for Lexitas and we think that it’s going to be the next frontier of personalized medicine and personalized clinical trial work in the ophthalmology community.
Thank you and have a great day
Additional Resources
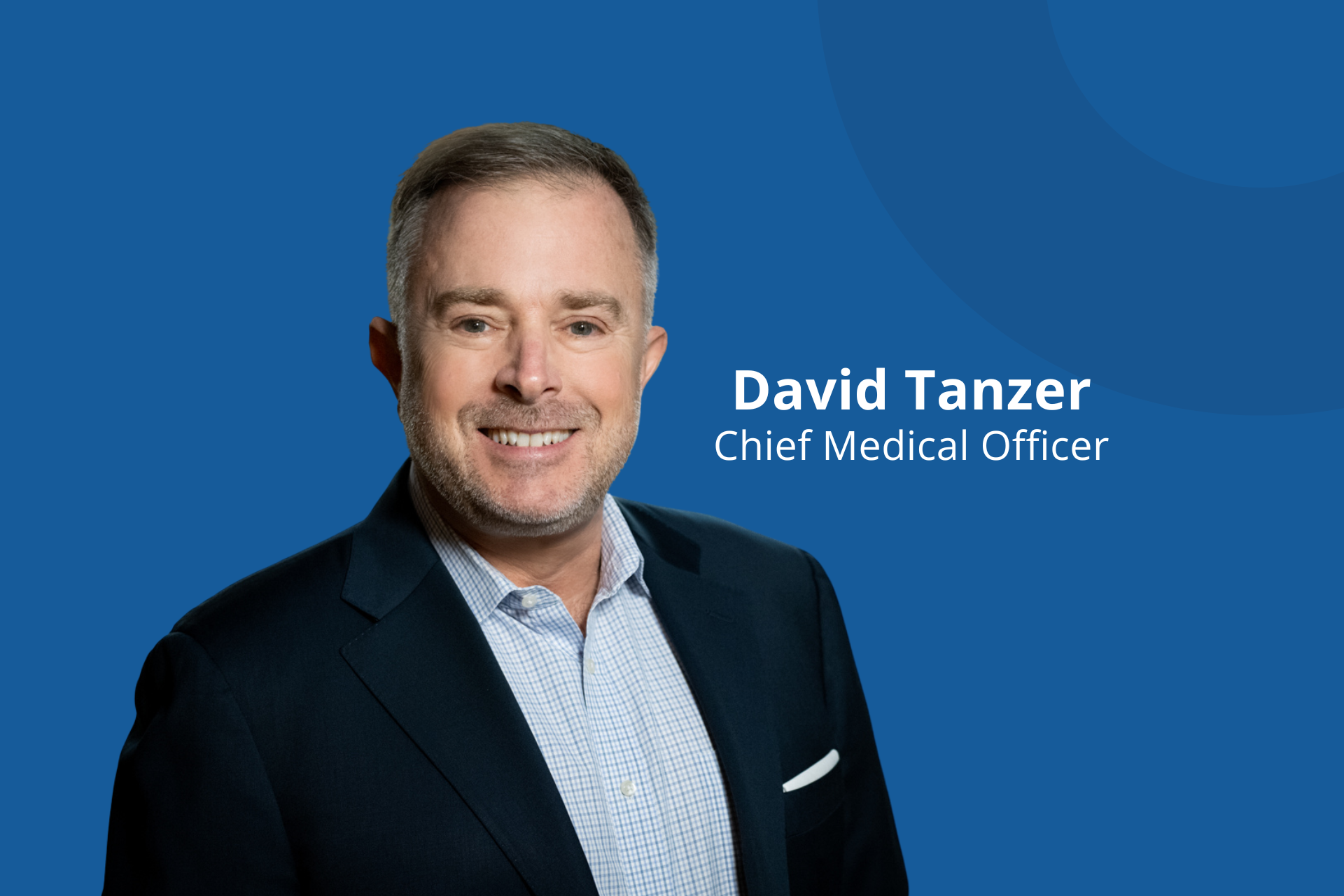
Lexitas Pharma Services Welcomes Dr. David Tanzer as Chief Medical Officer
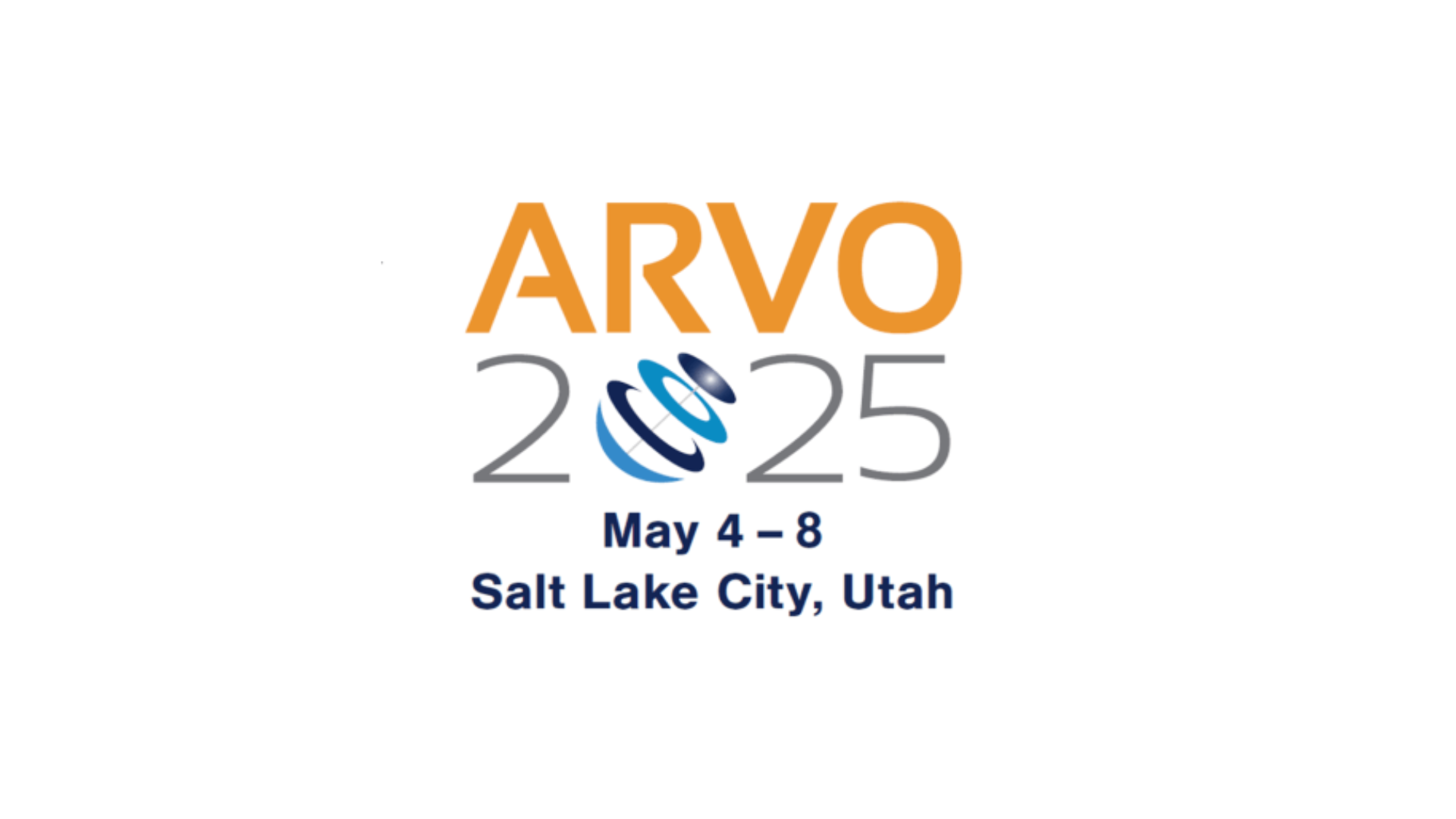
ARVO Annual Meeting
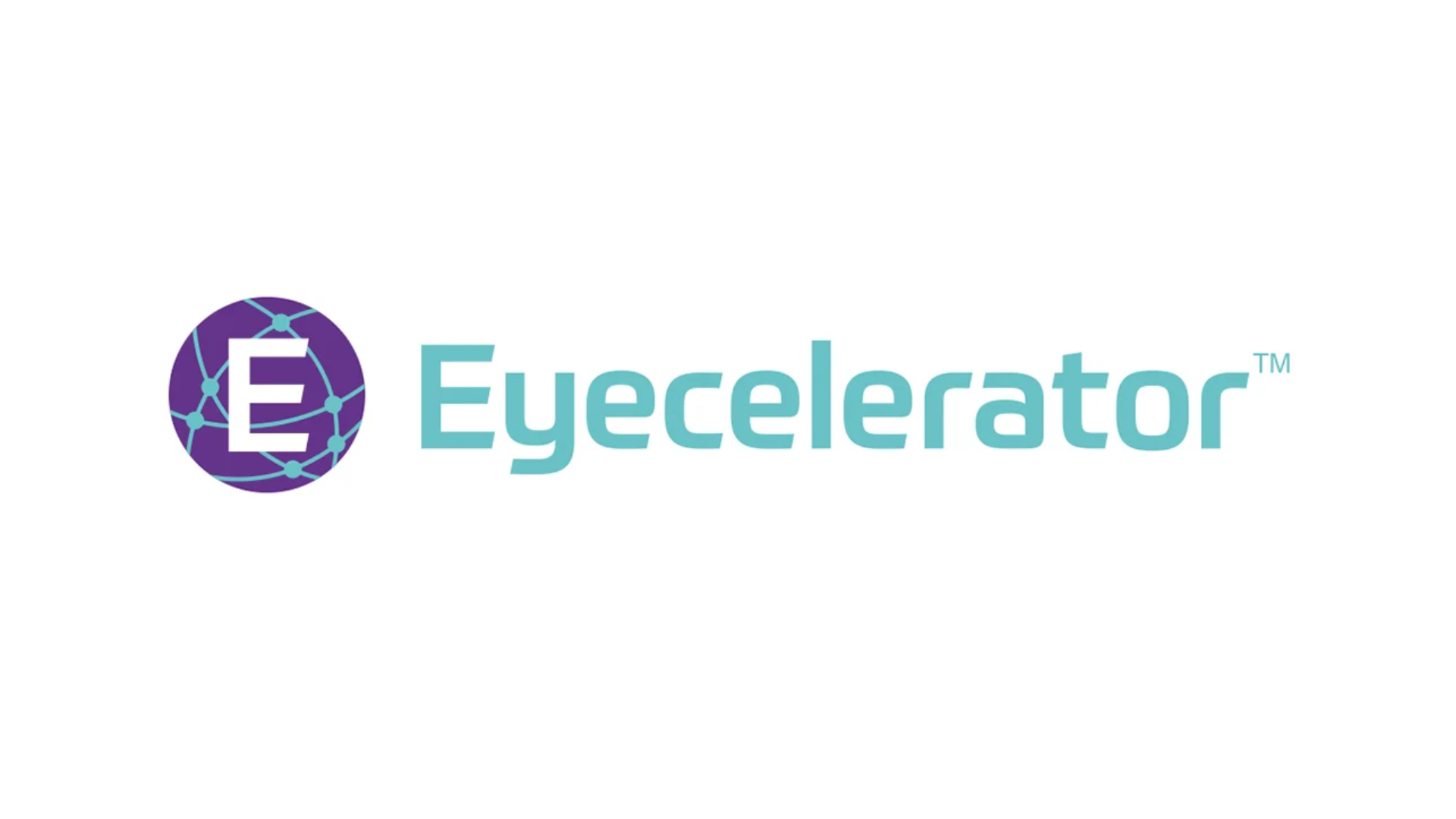
Eyecelerator @ Park City 2025
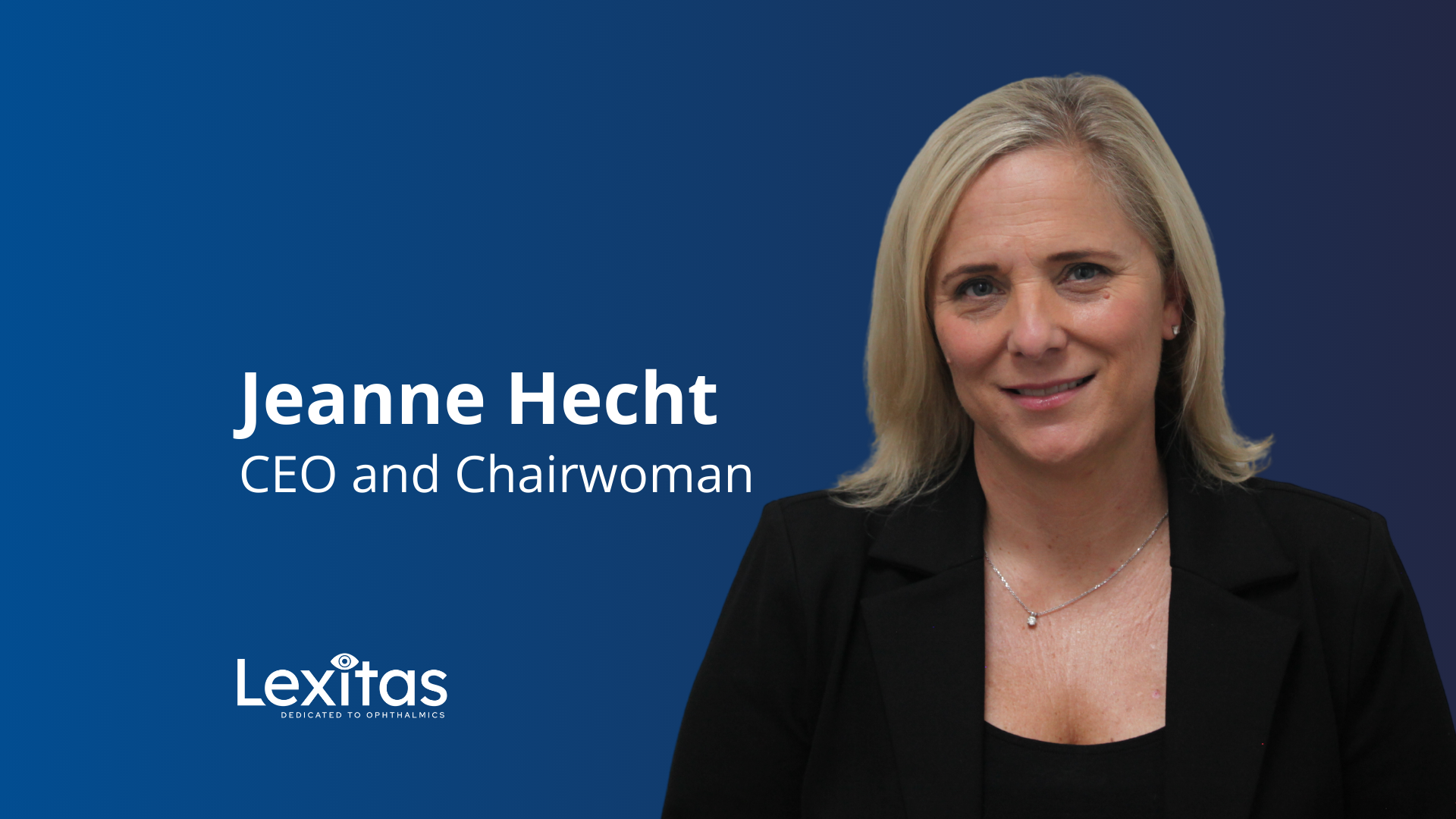
J.P. Morgan Takeaways with Jeanne Hecht
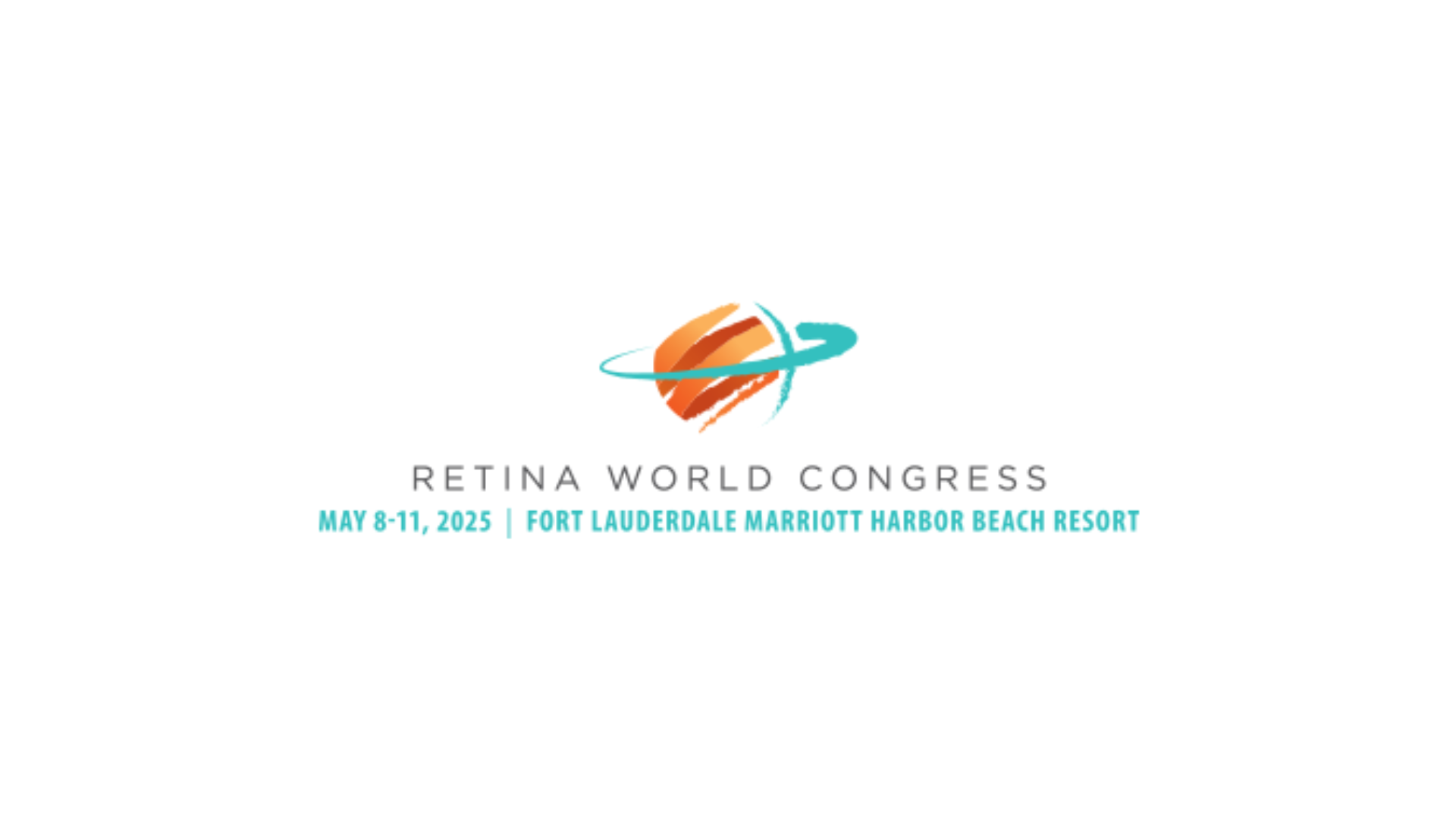
Retina World Congress
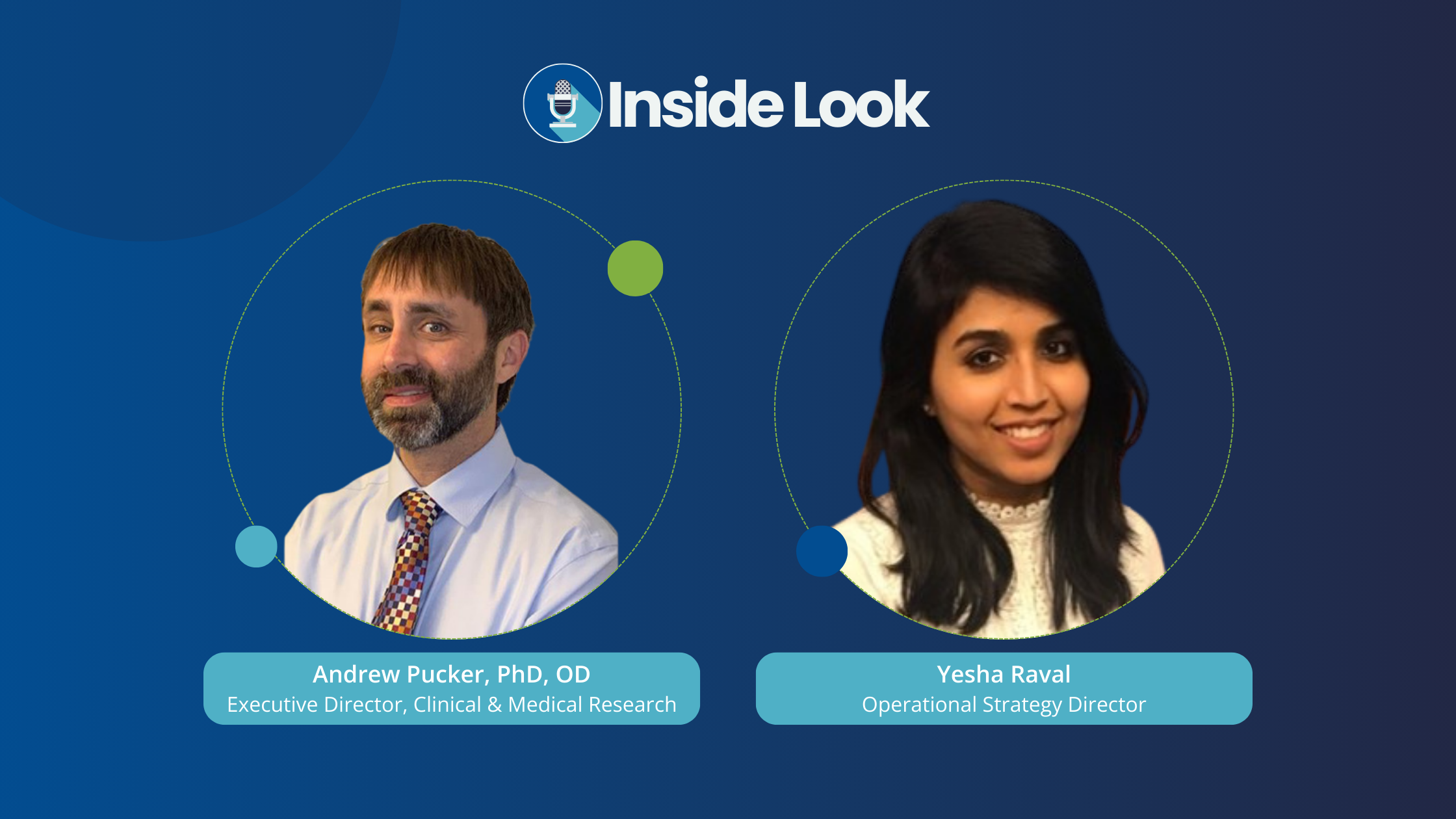